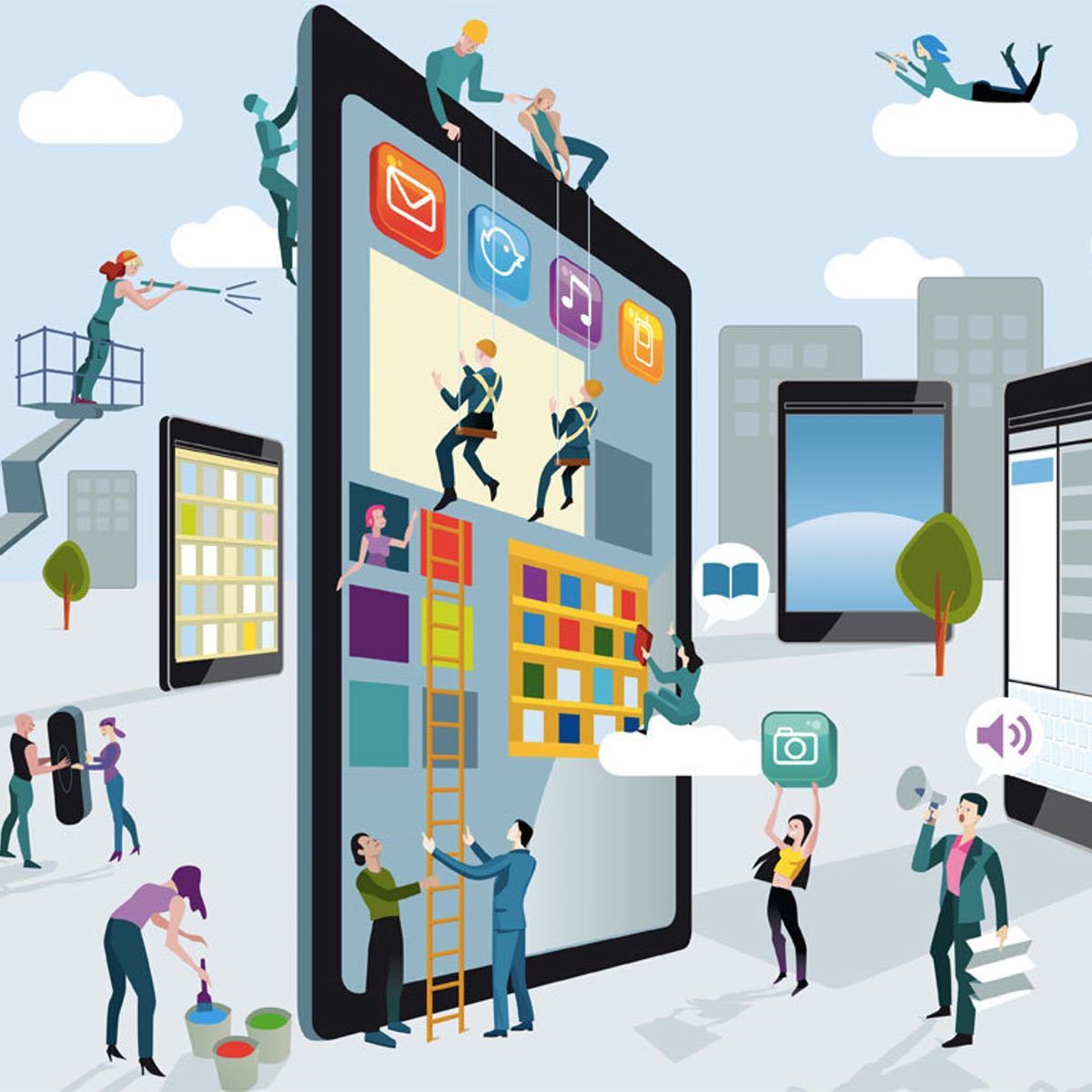
The Internet of Things, Big Data Analytics and KNIME
Posted on 15 March 2018 at 12:36 by Stefan Weingaertner
This interview was conducted in the run-up to KNIME Spring Summit 2016 within a series of interviews with data scientists invited to present at the Spring Summit.
Stefan Weingaertner, CEO of AdvancedAnalytics.Academy, talked about the drivers in his area of work and what his thoughts are on topics like data analytics, predictive analytics, the big data landscape and the internet of things.
KNIME: How did you get involved with the Internet of Things?
Stefan Weingaertner: My first contact with IoT was through a Smart Home project, which involved linking up end devices to the Internet and collating, monitoring and analyzing the flow of data and status information these devices transmitted. The focus of the project was to analyze the reciprocal effects in the usage of the end devices and the potential ways of optimizing consumption of energy; a smart meter measured the devices from the different households every 30 minutes.
KNIME: An example of a classic Internet of Things project?
Stefan Weingaertner: There are masses of service offerings and solutions in the Industrial Internet of Things. On the one hand we want to link up production machines and their equipment, but we also want to teach the "things" that are part of the Industrial Internet how to be smart. These production machines are capable of generating huge flows of data that need to be managed and analyzed. All the technologies inherent in IoT, big data, machine learning and cloud computing need to be combined in order to properly realize the intelligent work pieces and Smart Factories propagated by Industry 4.0 initiatives.
KNIME: Why did you decide to apply KNIME to analyze data from the Internet of Things?
Stefan Weingaertner: KNIME represents a quick and easy method of acquiring and integrating various data sources. KNIME also offers a large number of its own analysis algorithms, ranging from statistics methods to complex machine learning algorithms and - via R and Python nodes - access to literally loads of other algorithms that enable me to process and analyze data of all kinds of structures. The generation of quick and in-depth insight into the data is just one of KNIME's major strengths. And the KNIME Spark Executor enables you to process truly huge volumes of data.
KNIME: The most appreciated KNIME feature?
Stefan Weingaertner: It's difficult to highlight the KNIME feature I like the most, as the unique thing about KNIME is the seamless interplay of native KNIME nodes, additional nodes such as R and Python and the many contributions from the KNIME community. No other platform offers such consistent implementation of its open architecture.
KNIME: Internet of Things and Big Data. Do we really need big data?
Stefan Weingaertner: It's important here to make clear what we really mean by big data. The term "big data" is frequently used for methods that have been used for over 20 years already - which is something I personally consider to be critical. I see big data particularly in terms of new technology advances over conventional business intelligence environments, which, are essentially based on relational database systems and the potential to parallel process humongous volumes of data in batches, in near-time or real-time mode. This is where proven analytics algorithms have to be adjusted in order to be able to calculate accurate results in parallel. Related to the Internet of Things, big data technologies have to be applied when traditional data processes are no longer able to handle data economically in a reasonable time.
KNIME: Do you have any advice for companies starting the journey into the IoT with KNIME?
Stefan Weingaertner: Companies should first of all check which IoT use cases are really relevant and which not. Then they have to see which data sources can be tapped into in order to implement the use cases. It is important here to implement volume indicators to ascertain the expected volume of data. The advantage of KNIME here is that you can use the same platform (KNIME Analytics Platform) for a variety of questions, depending on the expected volume of data, and implement the KNIME Spark Executor nodes when you want to process really huge data volumes efficiently.
KNIME: What is the biggest challenge in applying data analytics to the Internet of Things?
Stefan Weingaertner: That's easy - data management: the acquisition of the relevant data sources, the integration of these data sources and feature engineering to train and deploy accurate prediction and classification models from the prepared data.
Here not only data management but also analysis (simple statistics to complex machine learning methods) must be able to be executed in batches, in near-time or in real-time, depending on the matter in hand.
KNIME: Which role do you think data mining can play in the Internet of Things?
Stefan Weingaertner: Data mining is crucial to the analysis of IoT data. Let's take the application of data mining in the Industrial Internet of Things as an example. Currently, wasteland in terms of analytics, because there are basically no solutions in a position to acquire these data. Our partner DATATRONiQ is closing this gap by connecting machines and their equipment and processing and compressing the data in real-time. They create a unique industrial data universe in which the problems of data-driven status monitoring (predictive maintenance) and automated quality control can be implemented efficiently and accurately with analysis platforms such as KNIME. The data mining methods in KNIME can, for example, identify status-related machine anomalies and forecast the best possible moment in time for maintenance, or they can highlight the relations between cause and effect in returned products, which is simply not possible with conventional analysis technologies to the scope that is required. In short: the collation of IoT data does not make much sense if we're not able to analyze it properly. But there is another challenge: which are the right methodologies to process and analyze sensor-based data? It is a domain, where digital signal processing meets machine learning algorithms. To get started quickly AdvancedAnalytics.Academy offers a one-day kickstart training for IoT Analytics.